Publications
Point-VOS: Pointing Up Video Object Segmentation
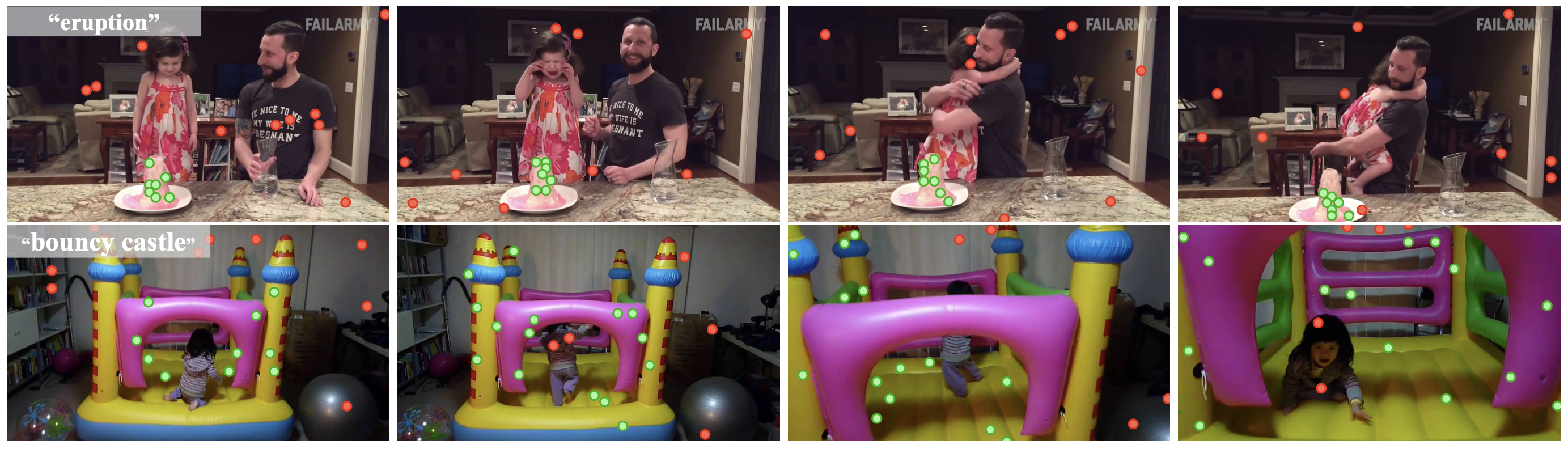
Current state-of-the-art Video Object Segmentation (VOS) methods rely on dense per-object mask annotations both during training and testing. This requires time-consuming and costly video annotation mechanisms. We propose a novel Point-VOS task with a spatio-temporally sparse point-wise annotation scheme that substantially reduces the annotation effort. We apply our annotation scheme to two large-scale video datasets with text descriptions and annotate over 19M points across 133K objects in 32K videos. Based on our annotations, we propose a new Point-VOS benchmark, and a corresponding point-based training mechanism, which we use to establish strong baseline results. We show that existing VOS methods can easily be adapted to leverage our point annotations during training, and can achieve results close to the fully-supervised performance when trained on pseudo-masks generated from these points. In addition, we show that our data can be used to improve models that connect vision and language, by evaluating it on the Video Narrative Grounding (VNG) task. We will make our code and annotations available at https://pointvos.github.io.
ControlRoom3D: Room Generation using Semantic Proxies
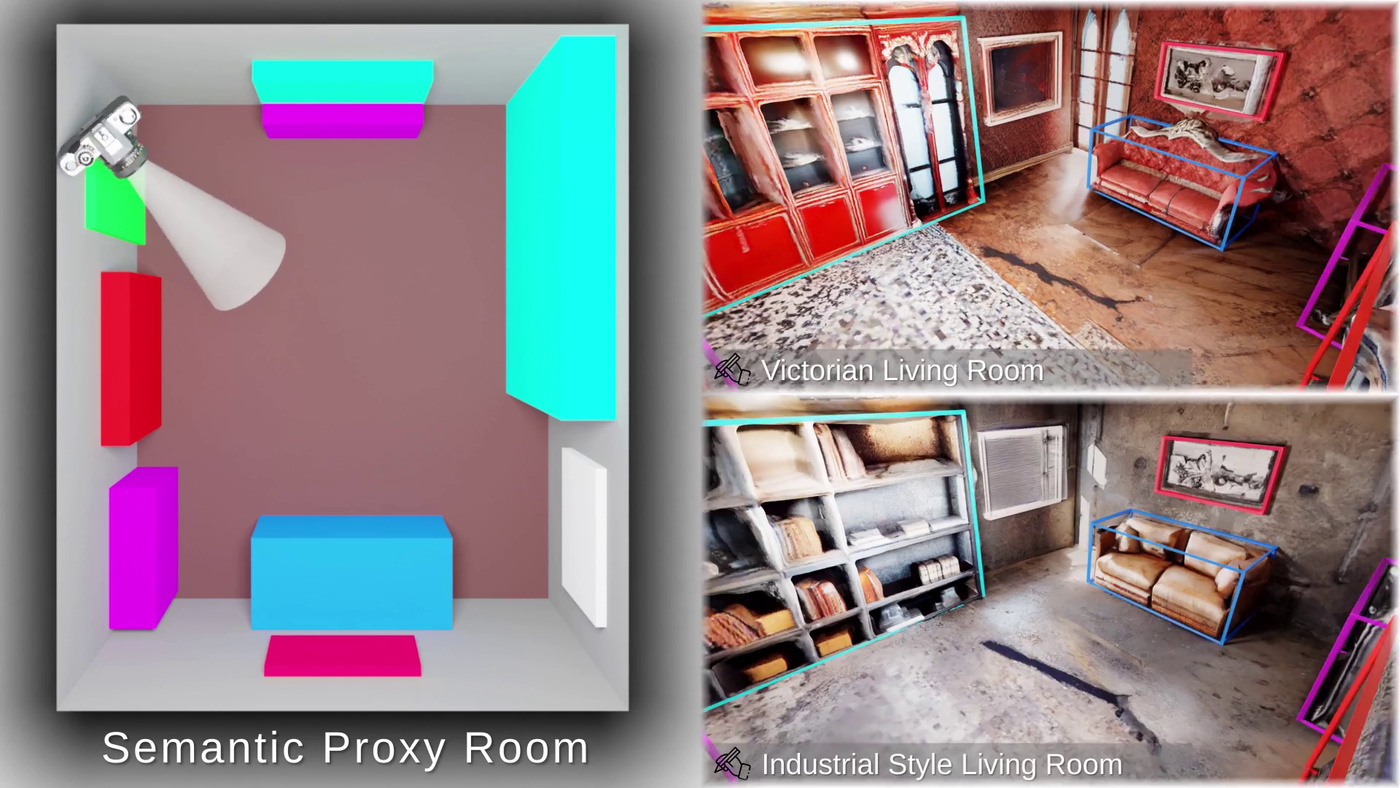
Manually creating 3D environments for AR/VR applications is a complex process requiring expert knowledge in 3D modeling software. Pioneering works facilitate this process by generating room meshes conditioned on textual style descriptions. Yet, many of these automatically generated 3D meshes do not adhere to typical room layouts, compromising their plausibility, e.g., by placing several beds in one bedroom. To address these challenges, we present ControlRoom3D, a novel method to generate high-quality room meshes. Central to our approach is a user-defined 3D semantic proxy room that outlines a rough room layout based on semantic bounding boxes and a textual description of the overall room style. Our key insight is that when rendered to 2D, this 3D representation provides valuable geometric and semantic information to control powerful 2D models to generate 3D consistent textures and geometry that aligns well with the proxy room. Backed up by an extensive study including quantitative metrics and qualitative user evaluations, our method generates diverse and globally plausible 3D room meshes, thus empowering users to design 3D rooms effortlessly without specialized knowledge.
@inproceedings{schult23controlroom3d,
author = {Schult, Jonas and Tsai, Sam and H\"ollein, Lukas and Wu, Bichen and Wang, Jialiang and Ma, Chih-Yao and Li, Kunpeng and Wang, Xiaofang and Wimbauer, Felix and He, Zijian and Zhang, Peizhao and Leibe, Bastian and Vajda, Peter and Hou, Ji},
title = {ControlRoom3D: Room Generation using Semantic Proxy Rooms},
booktitle = {IEEE Conference on Computer Vision and Pattern Recognition (CVPR)},
year = {2024},
}
Mask4Former: Mask Transformer for 4D Panoptic Segmentation
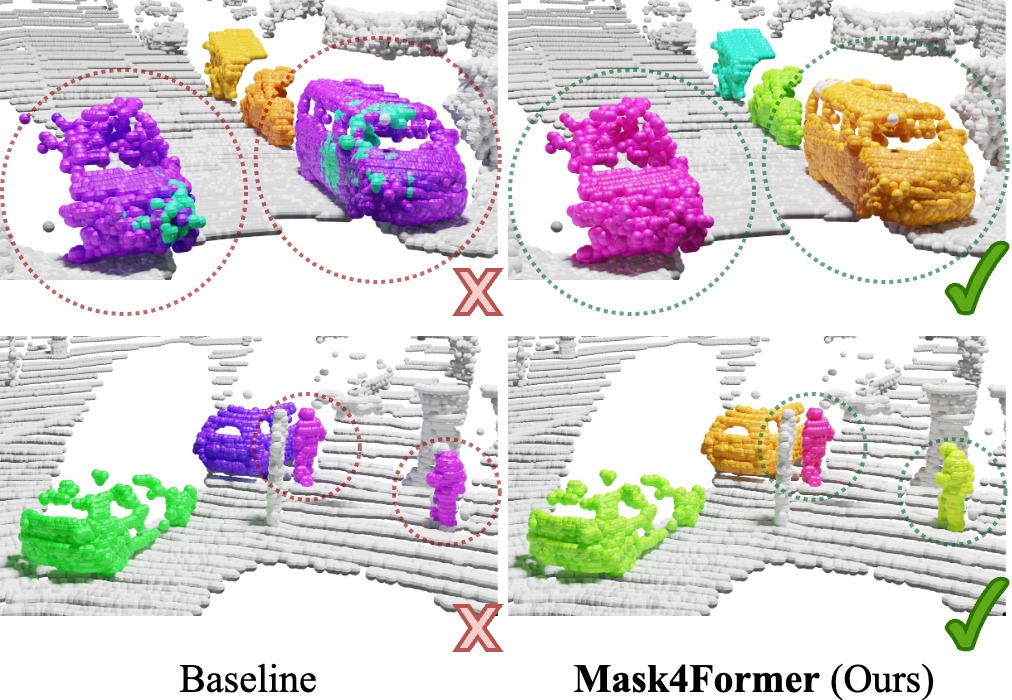
Accurately perceiving and tracking instances over time is essential for the decision-making processes of autonomous agents interacting safely in dynamic environments. With this intention, we propose Mask4Former for the challenging task of 4D panoptic segmentation of LiDAR point clouds.
Mask4Former is the first transformer-based approach unifying semantic instance segmentation and tracking of sparse and irregular sequences of 3D point clouds into a single joint model. Our model directly predicts semantic instances and their temporal associations without relying on hand-crafted non-learned association strategies such as probabilistic clustering or voting-based center prediction. Instead, Mask4Former introduces spatio-temporal instance queries that encode the semantic and geometric properties of each semantic tracklet in the sequence.
In an in-depth study, we find that promoting spatially compact instance predictions is critical as spatio-temporal instance queries tend to merge multiple semantically similar instances, even if they are spatially distant. To this end, we regress 6-DOF bounding box parameters from spatio-temporal instance queries, which are used as an auxiliary task to foster spatially compact predictions.
Mask4Former achieves a new state-of-the-art on the SemanticKITTI test set with a score of 68.4 LSTQ.
@inproceedings{yilmaz24mask4former,
title = {{Mask4Former: Mask Transformer for 4D Panoptic Segmentation}},
author = {Yilmaz, Kadir and Schult, Jonas and Nekrasov, Alexey and Leibe, Bastian},
booktitle = {International Conference on Robotics and Automation (ICRA)},
year = {2024}
}
AGILE3D: Attention Guided Interactive Multi-object 3D Segmentation
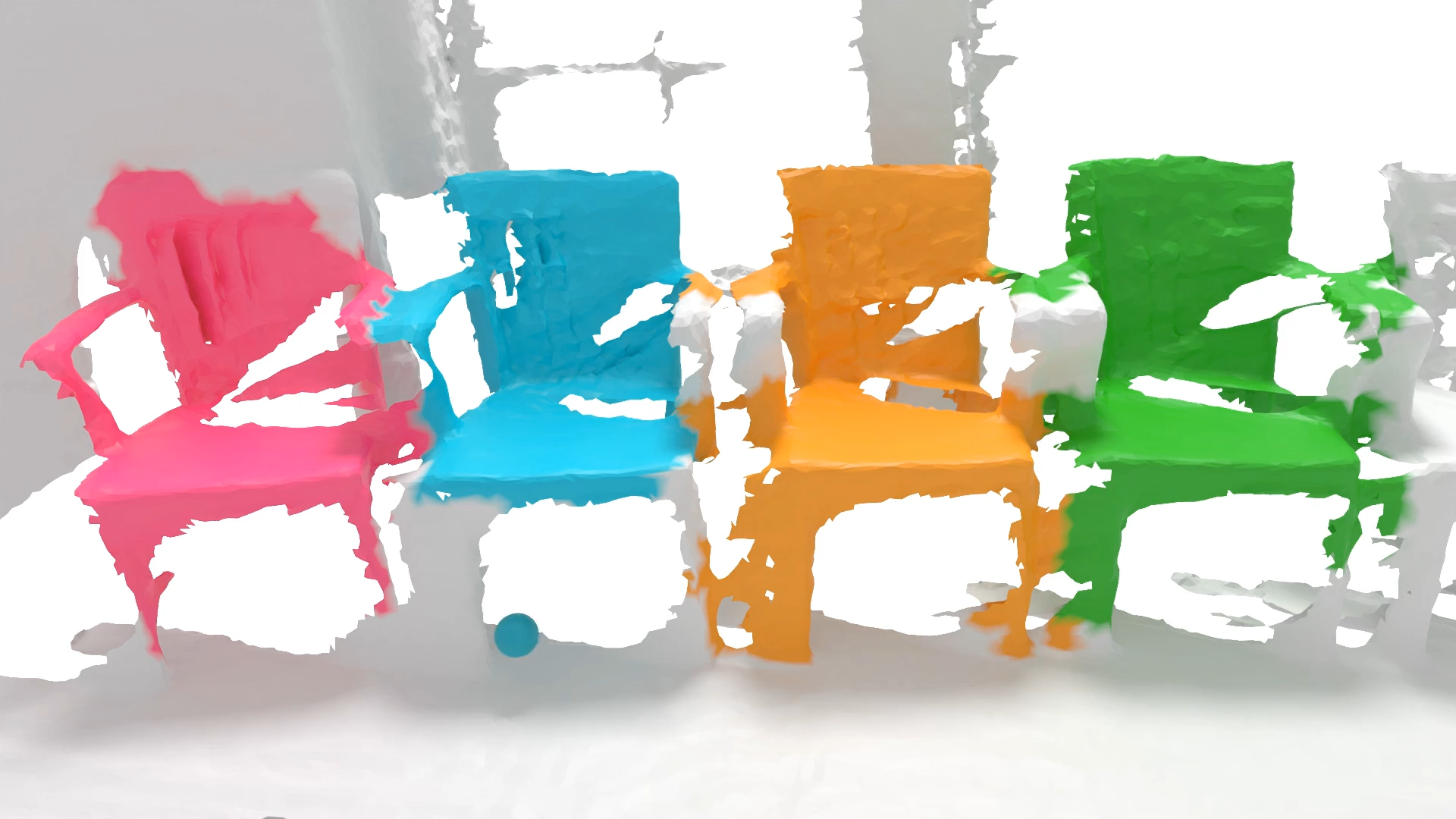
During interactive segmentation, a model and a user work together to delineate objects of interest in a 3D point cloud. In an iterative process, the model assigns each data point to an object (or the background), while the user corrects errors in the resulting segmentation and feeds them back into the model. The current best practice formulates the problem as binary classification and segments objects one at a time. The model expects the user to provide positive clicks to indicate regions wrongly assigned to the background and negative clicks on regions wrongly assigned to the object. Sequentially visiting objects is wasteful since it disregards synergies between objects: a positive click for a given object can, by definition, serve as a negative click for nearby objects. Moreover, a direct competition between adjacent objects can speed up the identification of their common boundary. We introduce AGILE3D, an efficient, attention-based model that (1) supports simultaneous segmentation of multiple 3D objects, (2) yields more accurate segmentation masks with fewer user clicks, and (3) offers faster inference. Our core idea is to encode user clicks as spatial-temporal queries and enable explicit interactions between click queries as well as between them and the 3D scene through a click attention module. Every time new clicks are added, we only need to run a lightweight decoder that produces updated segmentation masks. In experiments with four different 3D point cloud datasets, AGILE3D sets a new state-of-the-art. Moreover, we also verify its practicality in real-world setups with real user studies.
@inproceedings{yue2023agile3d,
title = {{AGILE3D: Attention Guided Interactive Multi-object 3D Segmentation}},
author = {Yue, Yuanwen and Mahadevan, Sabarinath and Schult, Jonas and Engelmann, Francis and Leibe, Bastian and Schindler, Konrad and Kontogianni, Theodora},
booktitle = {International Conference on Learning Representations (ICLR)},
year = {2024}
}
Wayfinding in Immersive Virtual Environments as Social Activity Supported by Virtual Agents
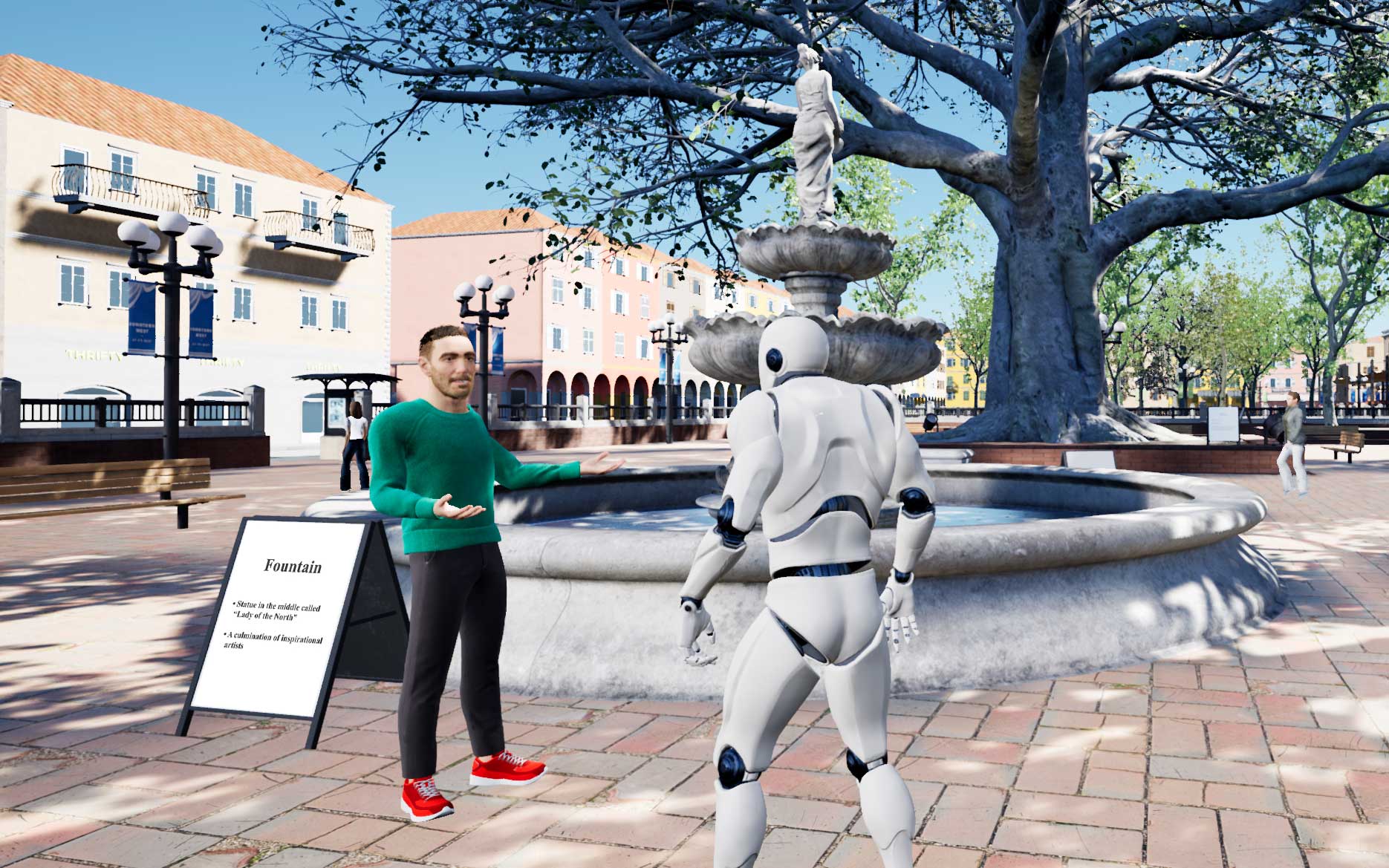
Effective navigation and interaction within immersive virtual environments rely on thorough scene exploration. Therefore, wayfinding is essential, assisting users in comprehending their surroundings, planning routes, and making informed decisions. Based on real-life observations, wayfinding is, thereby, not only a cognitive process but also a social activity profoundly influenced by the presence and behaviors of others. In virtual environments, these 'others' are virtual agents (VAs), defined as anthropomorphic computer-controlled characters, who enliven the environment and can serve as background characters or direct interaction partners. However, little research has been done to explore how to efficiently use VAs as social wayfinding support. In this paper, we aim to assess and contrast user experience, user comfort, and the acquisition of scene knowledge through a between-subjects study involving n = 60 participants across three distinct wayfinding conditions in one slightly populated urban environment: (i) unsupported wayfinding, (ii) strong social wayfinding using a virtual supporter who incorporates guiding and accompanying elements while directly impacting the participants' wayfinding decisions, and (iii) weak social wayfinding using flows of VAs that subtly influence the participants' wayfinding decisions by their locomotion behavior. Our work is the first to compare the impact of VAs' behavior in virtual reality on users' scene exploration, including spatial awareness, scene comprehension, and comfort. The results show the general utility of social wayfinding support, while underscoring the superiority of the strong type. Nevertheless, further exploration of weak social wayfinding as a promising technique is needed. Thus, our work contributes to the enhancement of VAs as advanced user interfaces, increasing user acceptance and usability.
@article{Boensch2024,
title={Wayfinding in Immersive Virtual Environments as Social Activity Supported by Virtual Agents},
author={B{\"o}nsch, Andrea and Ehret, Jonathan and Rupp, Daniel and Kuhlen, Torsten W.},
journal={Frontiers in Virtual Reality},
volume={4},
year={2024},
pages={1334795},
publisher={Frontiers},
doi={10.3389/frvir.2023.1334795}
}
VRScenarioBuilder: Free-Hand Immersive Authoring Tool for Scenario-based Testing of Automated Vehicles
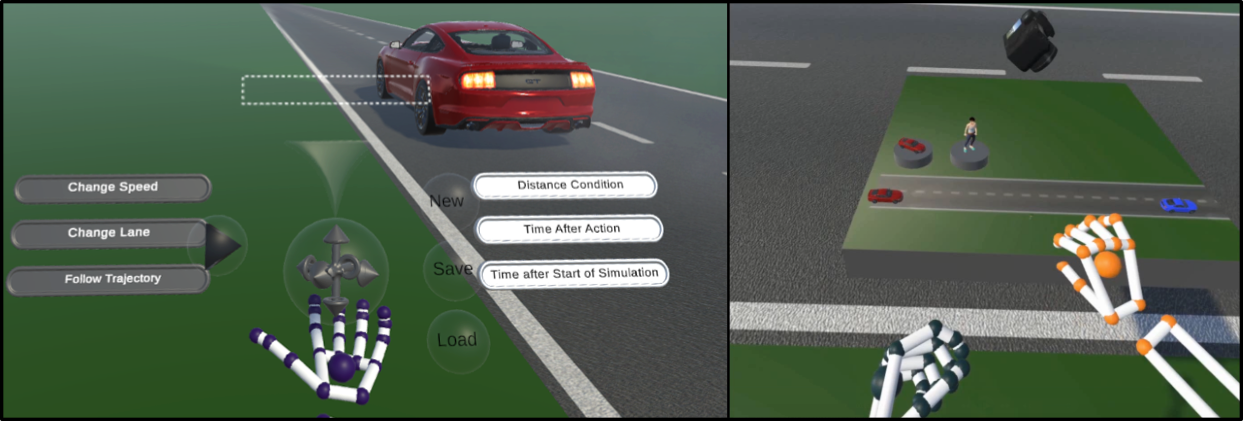
Virtual Reality has become an important medium in the automotive industry, providing engineers with a simulated platform to actively engage with and evaluate realistic driving scenarios for testing and validating automated vehicles. However, engineers are often restricted to using 2D desktop-based tools for designing driving scenarios, which can result in inefficiencies in the development and testing cycles. To this end, we present VRScenarioBuilder, an immersive authoring tool that enables engineers to create and modify dynamic driving scenarios directly in VR using free-hand interactions. Our tool features a natural user interface that enables users to create scenarios by using drag-and-drop building blocks. To evaluate the interface components and interactions, we conducted a user study with VR experts. Our findings highlight the effectiveness and potential improvements of our tool. We have further identified future research directions, such as exploring the spatial arrangement of the interface components and managing lengthy blocks.
On the Computation of User Placements for Virtual Formation Adjustments during Group Navigation
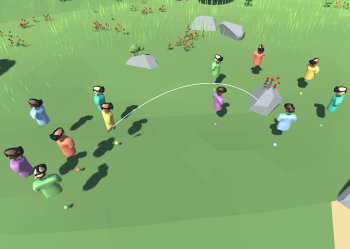
Several group navigation techniques enable a single navigator to control travel for all group members simultaneously in social virtual reality. A key aspect of this process is the ability to rearrange the group into a new formation to facilitate the joint observation of the scene or to avoid obstacles on the way. However, the question of how users should be distributed within the new formation to create an intuitive transition that minimizes disruptions of ongoing social activities is currently not explored. In this paper, we begin to close this gap by introducing four user placement strategies based on mathematical considerations, discussing their benefits and drawbacks, and sketching further novel ideas to approach this topic from different angles in future work. Our work, therefore, contributes to the overarching goal of making group interactions in social virtual reality more intuitive and comfortable for the involved users.
Try This for Size: Multi-Scale Teleportation in Immersive Virtual Reality
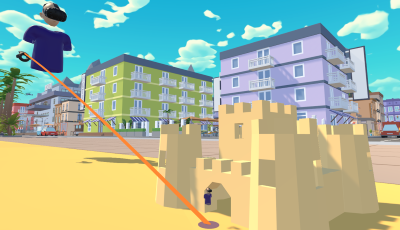
The ability of a user to adjust their own scale while traveling through virtual environments enables them to inspect tiny features being ant-sized and to gain an overview of the surroundings as a giant. While prior work has almost exclusively focused on steering-based interfaces for multi-scale travel, we present three novel teleportation-based techniques that avoid continuous motion flow to reduce the risk of cybersickness. Our approaches build on the extension of known teleportation workflows and suggest specifying scale adjustments either simultaneously with, as a connected second step after, or separately from the user’s new horizontal position. The results of a two-part user study with 30 participants indicate that the simultaneous and connected specification paradigms are both suitable candidates for effective and comfortable multi-scale teleportation with nuanced individual benefits. Scale specification as a separate mode, on the other hand, was considered less beneficial. We compare our findings to prior research and publish the executable of our user study to facilitate replication and further analyses.
StudyFramework: Comfortably Setting up and Conducting Factorial-Design Studies Using the Unreal Engine
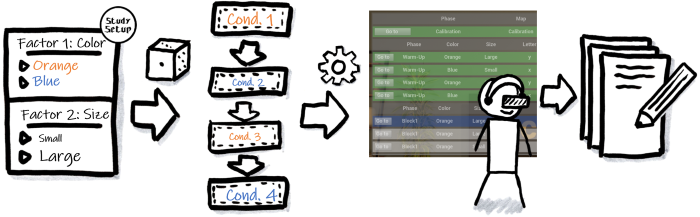
Setting up and conducting user studies is fundamental to virtual reality research. Yet, often these studies are developed from scratch, which is time-consuming and especially hard and error-prone for novice developers. In this paper, we introduce the StudyFramework, a framework specifically designed to streamline the setup and execution of factorial-design VR-based user studies within the Unreal Engine, significantly enhancing the overall process. We elucidate core concepts such as setup, randomization, the experimenter view, and logging. After utilizing our framework to set up and conduct their respective studies, 11 study developers provided valuable feedback through a structured questionnaire. This feedback, which was generally positive, highlighting its simplicity and usability, is discussed in detail.
@ InProceedings{Ehret2024a,
author={Ehret, Jonathan and Bönsch, Andrea and Fels, Janina and
Schlittmeier, Sabine J. and Kuhlen, Torsten W.},
booktitle={2024 IEEE Conference on Virtual Reality and 3D User Interfaces
Abstracts and Workshops (VRW): Workshop "Open Access Tools and Libraries for
Virtual Reality"},
title={StudyFramework: Comfortably Setting up and Conducting
Factorial-Design Studies Using the Unreal Engine},
year={2024}
}
Is Embodiment of Background Noise Sources a Necessity?
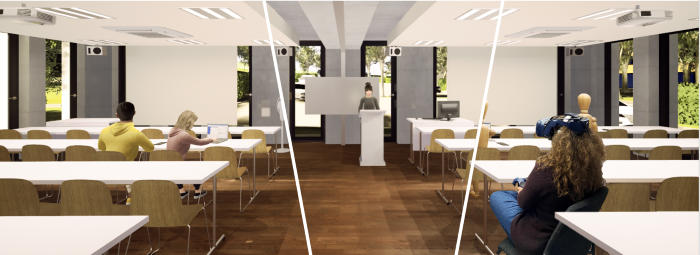
Exploring the synergy between visual and acoustic cues in virtual reality (VR) is crucial for elevating user engagement and perceived (social) presence. We present a study exploring the necessity and design impact of background sound source visualizations to guide the design of future soundscapes. To this end, we immersed n = 27 participants using a head-mounted display (HMD) within a virtual seminar room with six virtual peers and a virtual female professor. Participants engaged in a dual-task paradigm involving simultaneously listening to the professor and performing a secondary vibrotactile task, followed by recalling the heard speech content. We compared three types of background sound source visualizations in a within-subject design: no visualization, static visualization, and animated visualization. Participants’ subjective ratings indicate the importance of animated background sound source visualization for an optimal coherent audiovisual representation, particularly when embedding peer-emitted sounds. However, despite this subjective preference, audiovisual coherence did not affect participants’ performance in the dual-task paradigm measuring their listening effort.
@ InProceedings{Ehret2024b,
author={Ehret, Jonathan and Bönsch, Andrea and Schiller, Isabel S. and
Breuer, Carolin and Aspöck, Lukas and Fels, Janina and Schlittmeier, Sabine
J. and Kuhlen, Torsten W.},
booktitle={2024 IEEE Conference on Virtual Reality and 3D User Interfaces
Abstracts and Workshops (VRW): "Workshop on Virtual Humans and Crowds in
Immersive Environments (VHCIE)"},
title={Audiovisual Coherence: Is Embodiment of Background Noise Sources a
Necessity?},
year={2024}
}
Simulation of wire metal transfer in the cold metal transfer (CMT) variant of gas metal arc welding using the smoothed particle hydrodynamics (SPH) approach
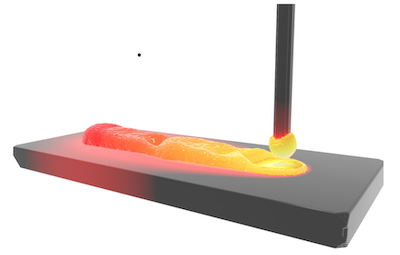
Cold metal transfer (CMT) is a variant of gas metal arc welding (GMAW) in which the molten metal of the wire is transferred to the weld pool mainly in the short-circuit phase. A special feature here is that the wire is retracted during this strongly controlled welding process. This allows precise and spatter-free formation of the weld seams with lower energy input. To simulate this process, a model based on the particle-based smoothed particle hydrodynamics (SPH) method is developed. This method provides a native solution for the mass and heat transfer. A simplified surrogate model was implemented as an arc heat source for welding simulation. This welding simulation model based on smoothed particle hydrodynamics method was augmented with surface effects, the Joule heating of the wire, and the effect of the electromagnetic forces. The model of metal transfer in the cold metal transfer process shows good qualitative agreement with real experiments.
@article{MWW+24,
author = {Mokrov, O. and Warkentin, S. and Westhofen, L. and Jeske, S. and Bender, J. and Sharma, R. and Reisgen, U.},
title = {Simulation of wire metal transfer in the cold metal transfer (CMT) variant of gas metal arc welding using the smoothed particle hydrodynamics (SPH) approach},
journal = {Materialwissenschaft und Werkstofftechnik},
volume = {55},
number = {1},
pages = {62-71},
keywords = {cold metal transfer (CMT), free surface deformation, gas metal arc welding (GMAW), simulation, smoothed particle hydrodynamics (SPH), geglätteter Partikel-basierter hydrodynamischer Ansatz (SPH), Kaltmetalltransfer (CMT), Metallschutzgasschweißens, Oberflächenverformung, Simulation},
doi = {https://doi.org/10.1002/mawe.202300166},
year = {2024}
}
Ray tracing method with implicit surface detection for smoothed particle hydrodynamics-based laser beam welding simulations
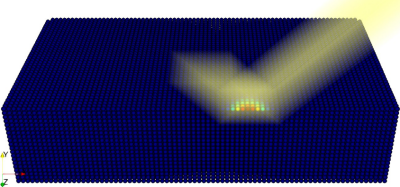
An important prerequisite for process simulations of laser beam welding is the accurate depiction of the surface energy distribution. This requires capturing the optical effects of the laser beam occurring at the free surface. In this work, a novel optics ray tracing scheme is proposed which can handle the reflection and absorption dynamics associated with laser beam welding. Showcasing the applicability of the approach, it is coupled with a novel surface detection algorithm based on smoothed particle hydrodynamics (SPH), which offers significant performance benefits over reconstruction-based methods. The results are compared to state-of-the-art experimental results in laser beam welding, for which an excellent correspondence in the case of the energy distributions inside capillaries is shown.
@article{WKB+24,
author = {Westhofen, L. and Kruska, J. and Bender, J. and Warkentin, S. and Mokrov, O. and Sharma, R. and Reisgen, U.},
title = {Ray tracing method with implicit surface detection for smoothed particle hydrodynamics-based laser beam welding simulations},
journal = {Materialwissenschaft und Werkstofftechnik},
volume = {55},
number = {1},
pages = {40-52},
keywords = {heat transfer, hydrodynamics, laser beam welding, ray optics, ray tracing, smoothed particle, geglättete Partikel, hydrodynamische, Laserstrahlschweißen, Strahloptik, Strahlverfolgung, Wärmetransfer},
doi = {https://doi.org/10.1002/mawe.202300161},
year = {2024}
}
Late-Breaking Report: VR-CrowdCraft: Coupling and Advancing Research in Pedestrian Dynamics and Social Virtual Reality
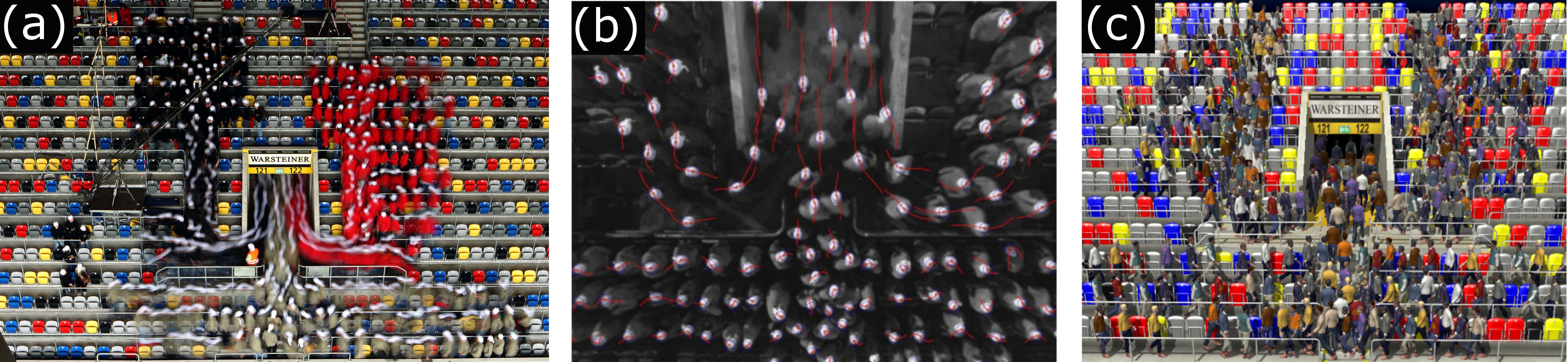
VR-CrowdCraft is a newly formed interdisciplinary initiative, dedicated to the convergence and advancement of two distinct yet interconnected research fields: pedestrian dynamics (PD) and social virtual reality (VR). The initiative aims to establish foundational workflows for a systematic integration of PD data obtained from real-life experiments, encompassing scenarios ranging from smaller clusters of approximately ten individuals to larger groups comprising several hundred pedestrians, into immersive virtual environments (IVEs), addressing the following two crucial goals: (1) Advancing pedestrian dynamic analysis and (2) Advancing virtual pedestrian behavior: authentic populated IVEs and new PD experiments. The LBR presentation will focus on goal 1.
Previous Year (2023)